|
ACCESS THE FULL ARTICLE
No SPIE Account? Create one
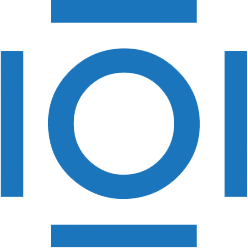
CITATIONS
Cited by 1 scholarly publication.
Sensors
Sensor performance
Object recognition
Visualization
Binary data
Machine vision
3D vision