|
ACCESS THE FULL ARTICLE
No SPIE Account? Create one
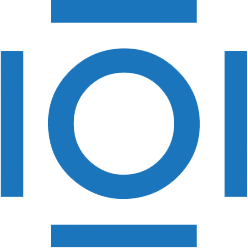
CITATIONS
Cited by 1 scholarly publication.
Feature extraction
System identification
Classification systems
Multimedia
Performance modeling
Speech recognition
Binary data