|
ACCESS THE FULL ARTICLE
No SPIE Account? Create one
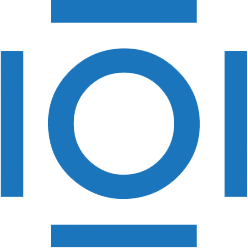
CITATIONS
Cited by 1 scholarly publication.
Tissues
Raman spectroscopy
Machine learning
Biopsy
Principal component analysis
Neurons
Diagnostics