|
ACCESS THE FULL ARTICLE
No SPIE Account? Create one
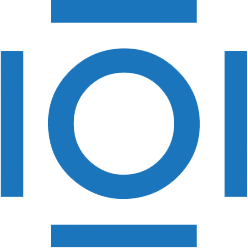
CITATIONS
Cited by 5 scholarly publications.
Infrared imaging
Video
Thermography
Infrared radiation
Infrared cameras
Sensors
Convolutional neural networks