|
ACCESS THE FULL ARTICLE
No SPIE Account? Create one
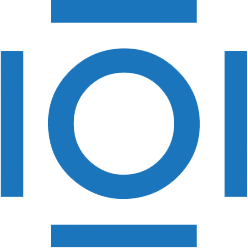
CITATIONS
Cited by 4 scholarly publications.
Synthetic aperture radar
Machine learning
Gallium nitride
Network architectures
Data modeling
Image processing
Detection and tracking algorithms