|
ACCESS THE FULL ARTICLE
No SPIE Account? Create one
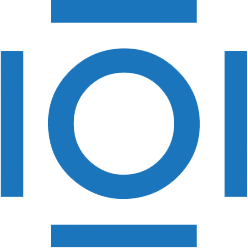
CITATIONS
Cited by 4 scholarly publications.
Unmanned aerial vehicles
Satellites
Data modeling
Agriculture
Stochastic processes
Clouds
Remote sensing