|
ACCESS THE FULL ARTICLE
No SPIE Account? Create one
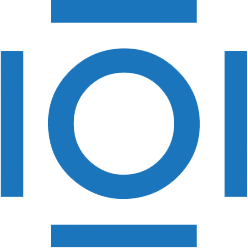
CITATIONS
Cited by 1 scholarly publication.
Convolution
Image classification
Hyperspectral imaging
Network architectures
Image sensors
Sensors
Neural networks