|
ACCESS THE FULL ARTICLE
No SPIE Account? Create one
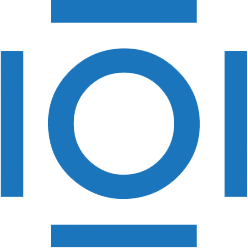
CITATIONS
Cited by 1 scholarly publication.
Signal to noise ratio
Interference (communication)
Signal detection
Matrices
Canonical correlation analysis
Signal processing
Environmental sensing