|
ACCESS THE FULL ARTICLE
No SPIE Account? Create one
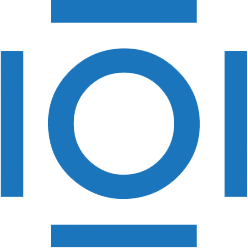
CITATIONS
Cited by 4 scholarly publications.
Neural networks
Visualization
Analog electronics
Fiber optics
Image visualization
Image intensifiers
Infrared imaging