|
ACCESS THE FULL ARTICLE
No SPIE Account? Create one
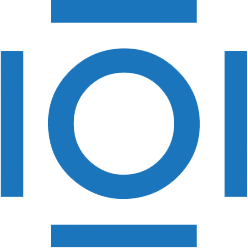
CITATIONS
Cited by 3 scholarly publications.
Atmospheric modeling
Wavefronts
Neural networks
Turbulence
Data modeling
Wavefront sensors
Atmospheric turbulence