|
ACCESS THE FULL ARTICLE
No SPIE Account? Create one
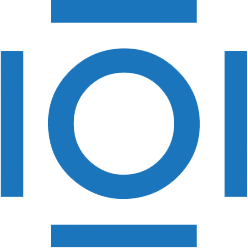
CITATIONS
Cited by 5 scholarly publications.
Genetics
Control systems
Satellites
Genetic algorithms
Sensors
Adaptive control
Neural networks