|
ACCESS THE FULL ARTICLE
No SPIE Account? Create one
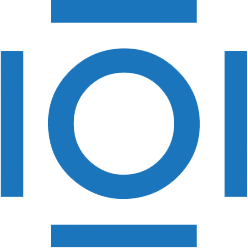
CITATIONS
Cited by 1 scholarly publication.
Target detection
Neural networks
Neurons
Feature extraction
Sensors
Land mines
Image fusion