|
ACCESS THE FULL ARTICLE
No SPIE Account? Create one
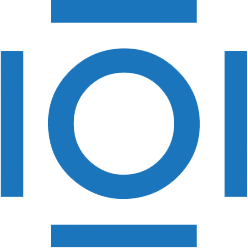
CITATIONS
Cited by 8 scholarly publications and 1 patent.
Control systems
Neural networks
Analog electronics
Actuators
Sensors
Systems modeling
Complex systems