|
ACCESS THE FULL ARTICLE
No SPIE Account? Create one
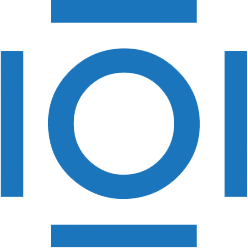
CITATIONS
Cited by 1 scholarly publication.
Image segmentation
Lung cancer
Neural networks
Neurons
Image processing
RGB color model
Image processing algorithms and systems