|
ACCESS THE FULL ARTICLE
No SPIE Account? Create one
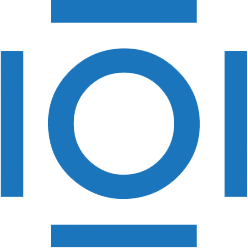
CITATIONS
Cited by 2 scholarly publications.
Statistical analysis
Vector spaces
Sensors
Error analysis
Sensor fusion
Data fusion
Fusion energy