|
ACCESS THE FULL ARTICLE
No SPIE Account? Create one
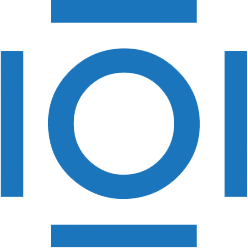
CITATIONS
Cited by 1 scholarly publication.
Cameras
Control systems
Calibration
Robot vision
Knowledge acquisition
Data acquisition
Signal processing