|
ACCESS THE FULL ARTICLE
No SPIE Account? Create one
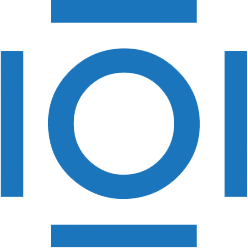
CITATIONS
Cited by 1 scholarly publication.
Neural networks
Lung
Chest imaging
Feature extraction
Image filtering
Pattern recognition
Lung cancer