|
ACCESS THE FULL ARTICLE
No SPIE Account? Create one
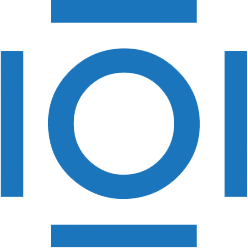
CITATIONS
Cited by 2 scholarly publications.
Neural networks
Mining
Land mines
Detection and tracking algorithms
Naval mines
Sensors
Acoustics