|
ACCESS THE FULL ARTICLE
No SPIE Account? Create one
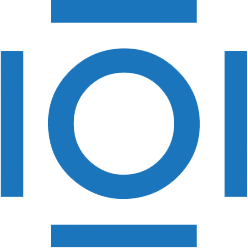
CITATIONS
Cited by 2 scholarly publications.
Principal component analysis
Image filtering
Denoising
Remote sensing
Image processing
Interference (communication)
Error analysis