|
ACCESS THE FULL ARTICLE
No SPIE Account? Create one
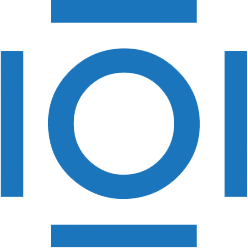
CITATIONS
Cited by 2 scholarly publications.
Neural networks
Principal component analysis
Data modeling
Model-based design
Statistical analysis
Earth observing sensors
Landsat