|
ACCESS THE FULL ARTICLE
No SPIE Account? Create one
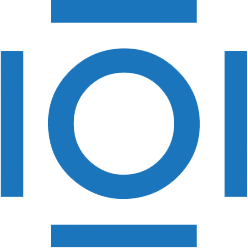
CITATIONS
Cited by 27 scholarly publications.
Digital filtering
Nonlinear filtering
Filtering (signal processing)
Motion models
Data modeling
Sensors
Electronic filtering