|
ACCESS THE FULL ARTICLE
No SPIE Account? Create one
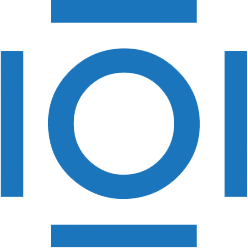
CITATIONS
Cited by 6 scholarly publications.
Image compression
Visualization
Visual compression
Visibility
Computer programming
Mammography
Visual process modeling