|
ACCESS THE FULL ARTICLE
No SPIE Account? Create one
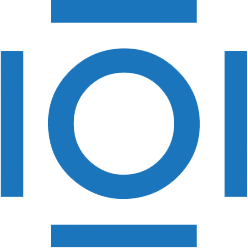
CITATIONS
Cited by 1 scholarly publication.
Logic
Data storage
Fuzzy logic
Computer programming
Data compression
Error analysis
Estimation theory