|
ACCESS THE FULL ARTICLE
No SPIE Account? Create one
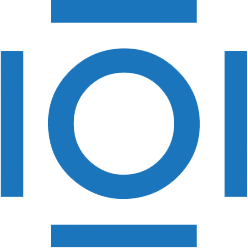
CITATIONS
Cited by 2 scholarly publications.
Galactic astronomy
Silver
Principal component analysis
Associative arrays
Astronomy
Feature extraction
Antimony