|
ACCESS THE FULL ARTICLE
No SPIE Account? Create one
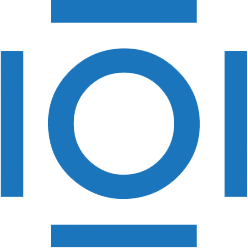
CITATIONS
Cited by 1 scholarly publication.
Wavelets
Denoising
Performance modeling
Statistical analysis
Image denoising
Wavelet transforms
Estimation theory