|
ACCESS THE FULL ARTICLE
No SPIE Account? Create one
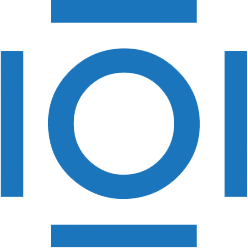
CITATIONS
Cited by 2 scholarly publications.
Ferroelectric LCDs
Facial recognition systems
Distance measurement
Principal component analysis
Performance modeling
Data modeling
Feature extraction