|
ACCESS THE FULL ARTICLE
No SPIE Account? Create one
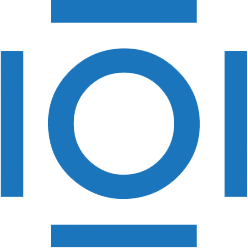
CITATIONS
Cited by 4 scholarly publications.
Image filtering
Optical filters
Neural networks
Hybrid optics
Nonlinear filtering
Distortion
Chlorine