|
ACCESS THE FULL ARTICLE
No SPIE Account? Create one
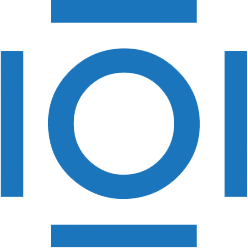
CITATIONS
Cited by 1 scholarly publication.
Diffusion
Particles
Systems modeling
Convolution
Bessel functions
Chemical reactions
Liquids