|
ACCESS THE FULL ARTICLE
No SPIE Account? Create one
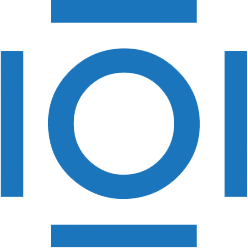
CITATIONS
Cited by 1 scholarly publication.
Neurons
Neural networks
Systems modeling
Mechanics
Stochastic processes
Thermodynamics
Data modeling