|
ACCESS THE FULL ARTICLE
No SPIE Account? Create one
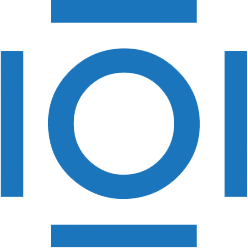
CITATIONS
Cited by 2 scholarly publications.
Infrared imaging
Statistical analysis
Error analysis
Automatic target recognition
Algorithm development
Virtual colonoscopy
Detection and tracking algorithms