|
ACCESS THE FULL ARTICLE
No SPIE Account? Create one
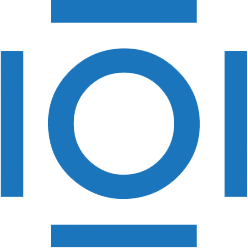
CITATIONS
Cited by 21 scholarly publications and 1 patent.
LIDAR
Expectation maximization algorithms
Data modeling
Buildings
Roads
Visualization
Airborne remote sensing