|
ACCESS THE FULL ARTICLE
No SPIE Account? Create one
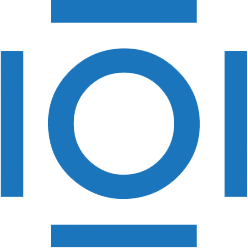
CITATIONS
Cited by 20 scholarly publications.
Computer intrusion detection
Evolutionary algorithms
Systems modeling
Binary data
Data modeling
Detection and tracking algorithms
Machine learning