|
ACCESS THE FULL ARTICLE
No SPIE Account? Create one
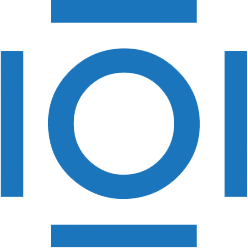
CITATIONS
Cited by 1 scholarly publication.
Matrices
Data mining
Biological research
Data processing
Image classification
Image processing
Image understanding