|
ACCESS THE FULL ARTICLE
No SPIE Account? Create one
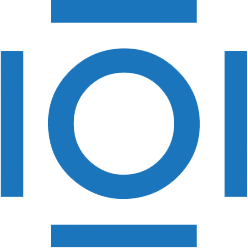
CITATIONS
Cited by 3 scholarly publications.
Time-frequency analysis
Sensors
Associative arrays
Feature extraction
Structural health monitoring
Statistical analysis
Aluminum