|
ACCESS THE FULL ARTICLE
No SPIE Account? Create one
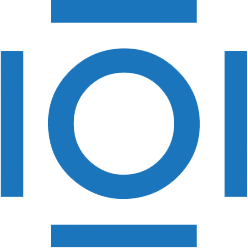
CITATIONS
Cited by 2 scholarly publications.
Sensors
Hyperspectral imaging
Mahalanobis distance
Remote sensing
Algorithm development
Data modeling
Factor analysis