|
ACCESS THE FULL ARTICLE
No SPIE Account? Create one
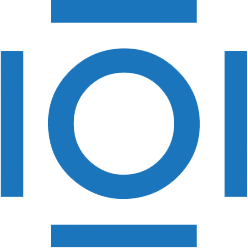
CITATIONS
Cited by 36 scholarly publications and 1 patent.
Denoising
Digital filtering
Interference (communication)
Filtering (signal processing)
Modulation
Optical filters
Electronic filtering