|
ACCESS THE FULL ARTICLE
No SPIE Account? Create one
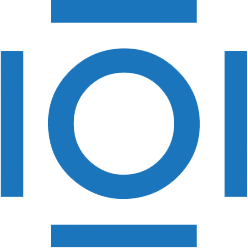
CITATIONS
Cited by 16 scholarly publications.
Feature extraction
Video
Detection and tracking algorithms
Neural networks
Neurons
Motion estimation
Optical flow