|
ACCESS THE FULL ARTICLE
No SPIE Account? Create one
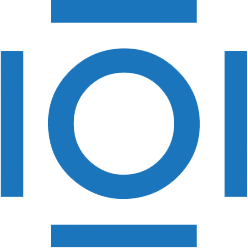
CITATIONS
Cited by 6 scholarly publications.
Databases
Error analysis
Machine learning
Distortion
Intelligence systems
Classification systems
Computing systems