|
ACCESS THE FULL ARTICLE
No SPIE Account? Create one
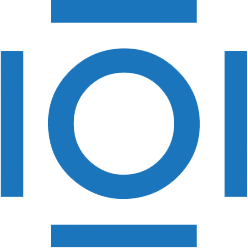
CITATIONS
Cited by 2 scholarly publications.
Associative arrays
Hyperspectral imaging
Image compression
Very large scale integration
JPEG2000
Stochastic processes
Optimization (mathematics)