|
ACCESS THE FULL ARTICLE
No SPIE Account? Create one
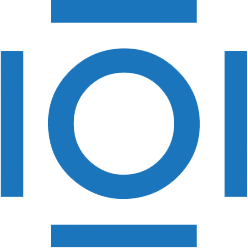
CITATIONS
Cited by 1 scholarly publication.
Vegetation
Remote sensing
Data modeling
Statistical modeling
Biochemistry
Reflectivity
Image classification