|
ACCESS THE FULL ARTICLE
No SPIE Account? Create one
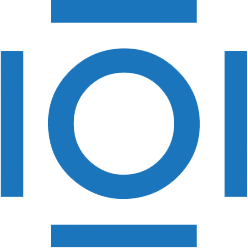
CITATIONS
Cited by 1 patent.
Optical lithography
Device simulation
Line width roughness
Transistors
Fabrication
Manufacturing
Visualization