|
ACCESS THE FULL ARTICLE
No SPIE Account? Create one
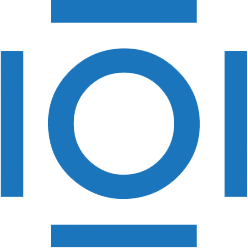
CITATIONS
Cited by 1 scholarly publication.
Sensors
Data modeling
Video
Systems modeling
Performance modeling
Statistical modeling
Visual process modeling