To resolve the problem of occlusion of the depth information of x-ray images and the detection of small-scale contraband in the detection of contraband objects, an improved prohibited item detection network has been proposed based on YOLOX. First, a material-aware atrous convolution module (MACM) is added to the feature pyramid network to enhance the model’s multiscale fusion and extraction ability for material information in x-ray image. Second, a spatial pyramid split attention mechanism (SPSA) is proposed to fuse spatial and channel attention for different scale spatial information features. Finally, CutMix data augmentation strategy is adopted to improve the robustness of the model. The overall performance identification experiments were conducted on the publicly available OPIXray dataset. The average accuracy (mean average precision, mAP) of the method is 93.10%. Compared with the baseline model YOLOX, the mAP is improved by 3.25%. The experimental results show that our method achieves state-of-the-art detection accuracy compared with existing methods. |
ACCESS THE FULL ARTICLE
No SPIE Account? Create one
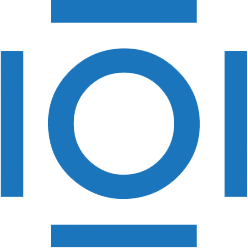
CITATIONS
Cited by 1 scholarly publication.
Convolution
X-rays
Object detection
X-ray imaging
Image enhancement
Data modeling
Feature extraction