|
ACCESS THE FULL ARTICLE
No SPIE Account? Create one
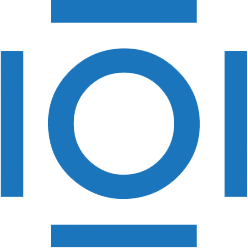
CITATIONS
Cited by 4 scholarly publications.
Autoregressive models
Detection and tracking algorithms
Computer simulations
Algorithm development
Earthquakes
Data modeling
Feature extraction