|
ACCESS THE FULL ARTICLE
No SPIE Account? Create one
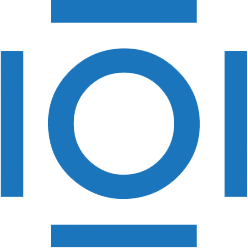
CITATIONS
Cited by 11 scholarly publications.
Image segmentation
Solid modeling
CAD systems
Tumor growth modeling
Computer aided design
Magnetorheological finishing
Prostate