|
ACCESS THE FULL ARTICLE
No SPIE Account? Create one
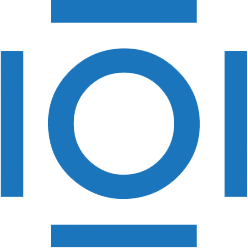
CITATIONS
Cited by 1 scholarly publication.
Copper
Sensors
Image processing
Computing systems
Detection and tracking algorithms
Edge detection
Machine vision