|
ACCESS THE FULL ARTICLE
No SPIE Account? Create one
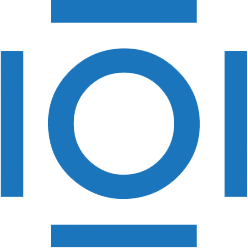
CITATIONS
Cited by 1 scholarly publication.
Associative arrays
Detection and tracking algorithms
Optical tracking
Algorithm development
Knowledge management
Lithium
Control systems