|
ACCESS THE FULL ARTICLE
No SPIE Account? Create one
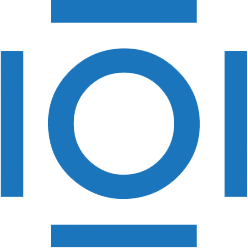
CITATIONS
Cited by 4 scholarly publications.
Neurons
Neural networks
Spatial light modulators
Classification systems
Data modeling
Mirrors
Detection and tracking algorithms