|
ACCESS THE FULL ARTICLE
No SPIE Account? Create one
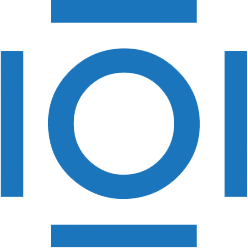
CITATIONS
Cited by 3 scholarly publications.
Image segmentation
Video
RGB color model
Image fusion
Active optics
Electroluminescent displays
Fourier transforms