|
ACCESS THE FULL ARTICLE
No SPIE Account? Create one
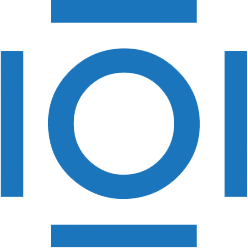
CITATIONS
Cited by 6 scholarly publications.
Hyperspectral imaging
Image classification
Feature extraction
Remote sensing
Image processing
Principal component analysis
Computer programming